Push Notifications – The Importance of A/B Testing and Using the Right Metrics
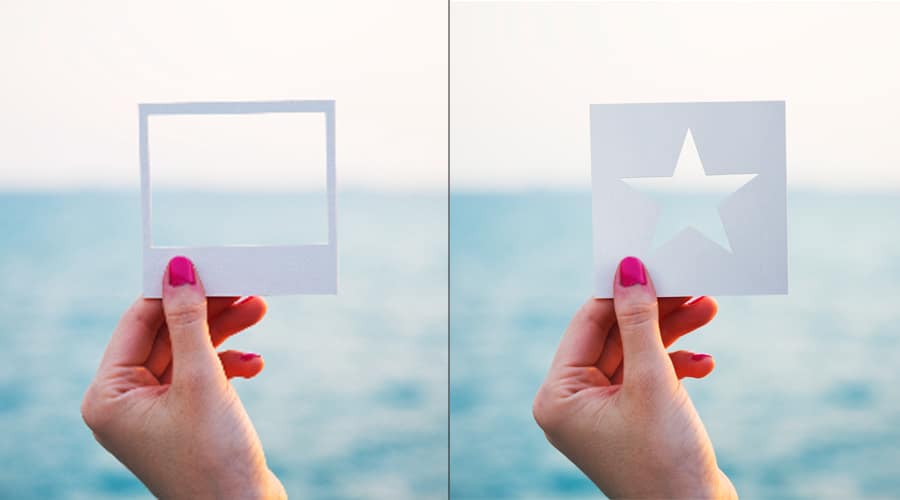
There are a ton of discussions about push notifications on mobile apps and the impacts they can cause on users. It’s clear that good push notifications can improve retention rates and keep users more engaged with apps…but are we prepared to understand the real impact of each message?
It’s common to expect numbers like increases in Conversion Rate, Retention or Sessions Per User, but there are other numbers, not so good to hear, that can be an indication of an incorrect Push Notifications strategy. It’s very important to identify the correct metrics, and create a model to do an effective follow-up day by day.
The first step is to define main objectives, like drive more Conversions, keep the app in the Users memory, increase the Sessions Per User, and so on. After that, we have to think about the better way to structure the message, followed by better moments to send, and finally send and do a follow-up based on the metrics defined earlier.
The problem with general metrics is: if you don’t define the correct aspects you will end up with only beautiful numbers to show in a presentation. These numbers will most likely be a reflection of vanity metrics. For example, it’s really important to have a high Open Rate, but what if we do not have good Conversion Rate? If we have a good Conversion Rate, but a higher Uninstall Rate, is the Push Notification strategy a good marketing tool?
That’s the reason we have to carefully define our metrics and the way we’ll track it.
What metrics should we track?
There are some common metrics for Push Notifications:
Open Rate/Click Rate: High Open Rates can explain attractive messages. Rich pushes, emojis, personalized data and behavior analysis can help a lot. The Open Rate can vary between operational systems and apps.
It’s only the beginning of the funnel, depending on your main objectives with the messages. For example if you are expecting an increase on Sign Up rate, the push open, alone, doesn’t help.
Conversion Rate: Here, we are talking about users that received a Push Notification and performed a Conversion Event, like a Sign-Up, a purchase or any other Event important for the app.
A good point about Conversion Rate is: if you received a message and didn’t open, was the Conversion actually impacted by the message you’ve received? Some marketers can argue that it isn’t a result of the message.
That said, what if you haven’t been using the app for the last 7 days and you saw the message while you were in a meeting? After the meeting you remembered that message, opened the app (without the push message itself), and did a Conversion? Maybe the same marketer can argue now that it was the impact of the message.
If we consider that a lot of Users look to their smartphones everytime something new arises, but not necessarily hold the phone and start to use it, maybe it’s correct to attribute this Conversion to a Push Notification.
Click-Through Conversion Rate: In this case, Click-Through Conversion Rates count only Conversions from Users who opened the message, representing the whole funnel. It’s a more accurate number, but certainly will be lower than the previous metric (ie only Conversion Rate).
Assuming we are using the metrics above, let’s examine the follow scenario:
In a blink of the eye we can assume that Push Notification 2 is better than Push Notification 1. However, maybe it’s not completely true.
Working with A/B testing
The example above is quite simple, and we not using troubles like the Revenue From Conversions and the cost to send each message (very important to calculate ROI).
We can provide one more information about these scenarios:
Push Notification 1 was sent on Monday morning, a really bad time for the current app
Push Notification 2 was sent on Friday night, a better conversion day part for the same app
Now, it’s not clear that the Push Notification 1 is the real winner. So, how to improve our analysis and conclusion about the results? The answer is: A/B Testing.
There are a lot of tools available on the market (take a look at Localytics, for example) which allow us to create A/B tests, providing almost the same environment for the variations. So, we can set up a test to validate the numbers. After that, imagine that we received the following results:
Now, we are seeing that variation A as a clear winner.
In some cases, is simply impossible to compare different messages without the same environment conditions. For my current apps, for example, sometimes we have some conversations like:
Marketer: Let’s try to compare the current result with the same day on last week.
Analyst: Doesn’t work, because we had a holiday period, with higher Conversion Rate, last week.
Marketer: OK, let’s compare with the same day on last month.
Analyst: Doesn’t work, because it was on a different day of the week.
Marketer: OMG, then let’s compare then the whole result from this month with the last month.
Analyst: Doesn’t work, either, because last month was December and the holidays will break the analysis.
Marketer: (Pulls hair out and runs away, crying.)
To solve it, always use A/B Testing tools to correctly evaluate the results of each message.
Once you have the correct metrics and the A/B test structure in place, it’s time to validate each hypothesis and keep the improvements on the messages going like a PDCA cycle. If special deals are driving higher Open Rates, but not Conversions, maybe test with cheaper deals or higher discounts. If the Open Rate of simple messages are not so good, try with Rich Messages…and so on. You can always create an A/B test to discover new opportunities, with the correct metrics.